🟣 AI Readiness Series
Maximizing AI Value through Effective Data Management and Integration
Written by
Faizan Hussain, Senior Manager
Published
8.9.2023
Products mentioned
Data Analytics
Artificial Intelligence (AI) has become a game-changer for businesses worldwide, offering unparalleled opportunities to extract value from data and address complex challenges. To fully leverage AI’s potential, organizations must define clear use cases and objectives, assess data availability and quality, and implement effective data collection and integration strategies. In this blog post, we will explore how these crucial components work together to unlock the true power of AI and drive informed decision-making.
Defining AI Use Cases and Objectives for Maximum Impact
The first step in leveraging AI effectively is to identify the specific business problem or opportunity that you aim to address. Whether it is streamlining operational processes, enhancing customer experiences, optimizing resource allocation, or predicting market trends, it is essential to pinpoint the use case that aligns with your organization’s strategic goals. Defining the use case sets the context for data collection, analysis, and model development, ensuring that efforts are concentrated on the areas that will provide the most significant impact.
Once the use case is established, the next step is to set clear objectives for the AI project. Objectives outline the desired outcomes and define the metrics that will measure success. They help to focus efforts, guide decision-making, and monitor progress throughout the project lifecycle. Objectives should be specific, measurable, achievable, relevant, and time-bound (SMART), ensuring that they are realistic and attainable within the given constraints.
With the use case and objectives defined, the focus shifts to data preparation. Data is the lifeblood of AI systems, and the quality, relevance, and diversity of data play a critical role in the accuracy and effectiveness of AI models. By aligning data preparation efforts with the AI goals, businesses can ensure that the collected data variables are relevant and comprehensive enough to address the defined use case and objectives.
Assessing Data Availability and Quality for AI-Readiness
To harness the power of AI effectively, it is essential to identify the data sources that contain the relevant information required to address the AI use case. This involves understanding the nature of the problem or opportunity at hand and determining the types of data that can provide insights and support decision-making. By identifying and accessing the right data sources, organizations can lay the groundwork for meaningful analysis and model development.
Data quality is measured by six key dimensions: accuracy, completeness, consistency, timeliness, validity, and uniqueness.
Data completeness is a critical aspect of data quality. It refers to the extent to which the data captures all the necessary information required for the AI use case. During the assessment, it is important to evaluate whether the available data is comprehensive enough to address the objectives defined earlier. Are there any missing data points or gaps that may hinder accurate analysis? If so, organizations need to consider strategies to fill those gaps, such as data augmentation or seeking additional data sources.
The accuracy of data is paramount for reliable AI outcomes. During the assessment, organizations should scrutinize the data for any errors, inconsistencies, or outliers that may compromise the integrity of the analysis. This may involve data profiling, statistical analysis, or comparing data from multiple sources to identify discrepancies. By addressing data accuracy issues early on, organizations can ensure that their AI models are built on a solid foundation of reliable and trustworthy data.
Data reliability pertains to the trustworthiness and consistency of the data sources. It is crucial to evaluate the credibility and provenance of the data to ensure that it aligns with the organization’s standards and requirements. This assessment may involve understanding the data collection methods, data governance practices, and data validation processes employed by the data sources. Evaluating data reliability helps organizations mitigate the risk of basing decisions on flawed or biased data.
Based on the assessment results, organizations may need to undertake data cleansing and preprocessing steps to enhance the quality and usability of the data. Data cleansing involves identifying and resolving issues such as duplicate records, missing values, and inconsistent formatting. Preprocessing steps may include data normalization, feature engineering, and scaling, depending on the specific AI use case. By investing effort in data cleansing and preprocessing, organizations can optimize the performance and accuracy of their AI models.
The Power of Data Collection and Integration
Before embarking on the data collection and integration process, it is crucial to identify the relevant data sources. Once the relevant data sources have been identified, the next step is to collect data from these disparate sources. This process may involve using a combination of techniques such as data extraction, web scraping, or APIs (Application Programming Interfaces) to gather the required data. It is important to ensure the collected data is accurate, consistent, and adheres to any relevant data privacy regulations.
Data integration is the process of combining data from different sources into a unified repository or data warehouse. By consolidating data into a single location, organizations can eliminate data silos that often hinder comprehensive analysis. Siloed data is scattered across different systems or departments, making it difficult to gain a holistic view of the organization’s operations. Data integration allows for a holistic approach to data analysis, enabling cross-functional insights and fostering collaboration among teams. Data integration offers numerous benefits, including:
- Comprehensive analysis: Leveraging integrated data for deeper insights and decision-making
- Enhanced data quality: Ensuring reliable and trustworthy data through integration
- Real-time insights: Responding quickly to market trends and opportunities with timely data
- Streamlined reporting: Automating reporting processes for efficient information dissemination
Data Governance for Ethical Data Handling
While data collection and integration offer numerous benefits, there are challenges that organizations must address:
- Data Governance: Establishing data governance policies and procedures is crucial to ensure data privacy, security, and compliance. Organizations need to define roles, responsibilities, and access controls to protect sensitive data and ensure ethical data handling practices.
- Data Compatibility: Data collected from various sources may have different formats, structures, or standards. Ensuring compatibility and standardization during the integration process is essential to maintain data integrity and facilitate seamless analysis.
- Scalability: As data volumes grow, organizations need to ensure their data integration processes can handle increasing data loads efficiently. Scalable infrastructure and data integration technologies are necessary to support the expanding needs of the organization.
The Roadmap to AI-Ready Data
Defining AI use cases, assessing data quality, and embracing integration are essential pillars of successful AI implementation. Organizations that strategically combine these aspects can unlock the true potential of AI, making informed decisions, identifying opportunities, and gaining a competitive edge in the data-driven era.
To help you navigate the complexities of preparing your data for AI, OneSix has authored a comprehensive roadmap to AI-ready data. Our goal is to empower organizations with the knowledge and strategies needed to modernize their data platforms and tools, ensuring that their data is optimized for AI applications.
Read our step-by-step guide for a deep understanding of the initiatives required to develop a modern data strategy that drives business results.
More from our AI Readiness series
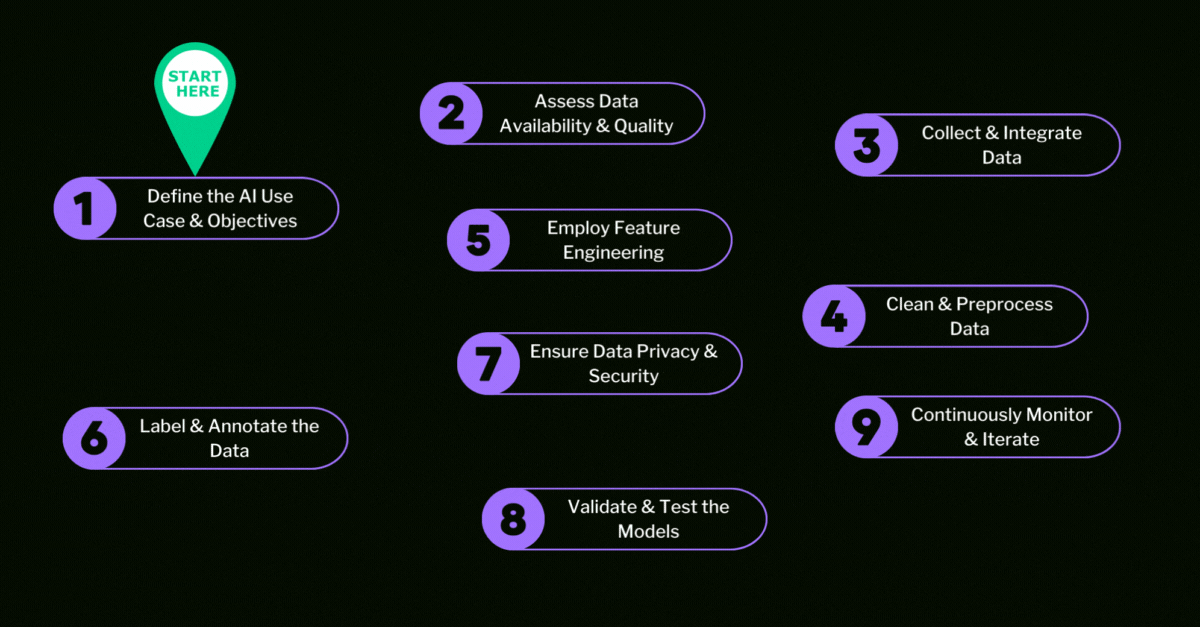
Unlocking the Power of AI: The Roadmap to AI-Ready Data
Guide
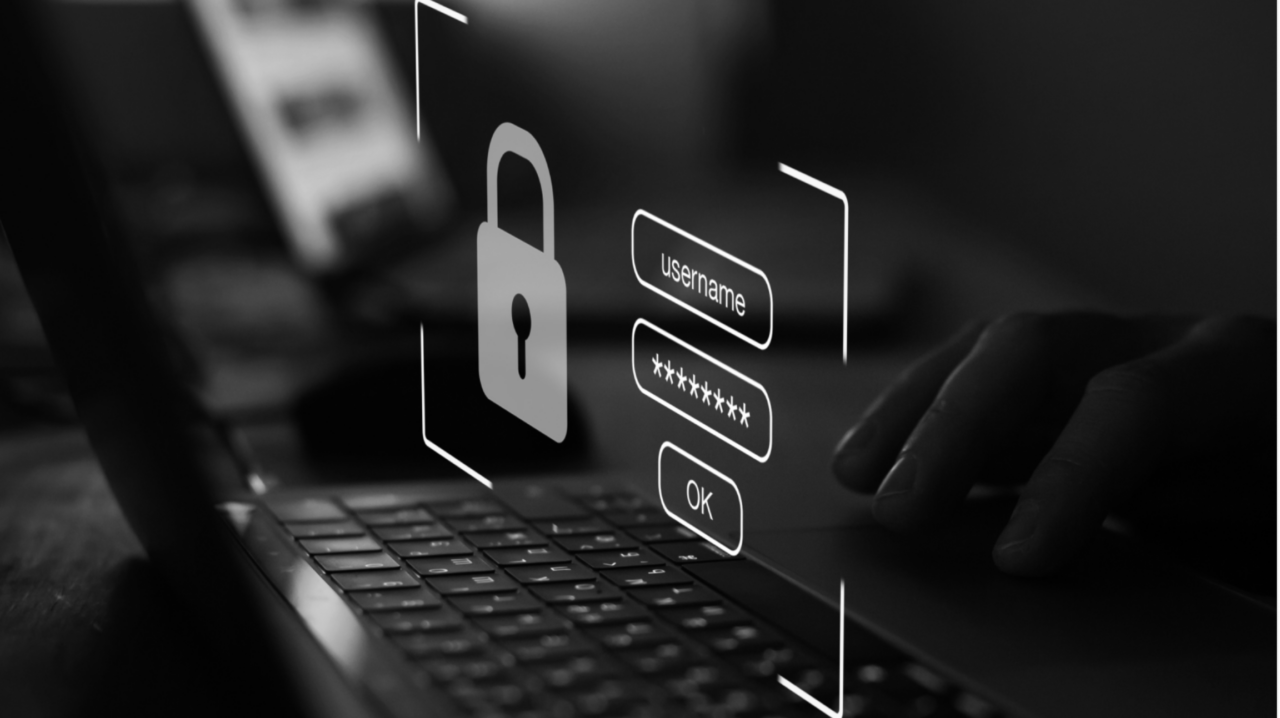
Ensuring AI Excellence: Data Privacy/Security and Model Validation
Blog
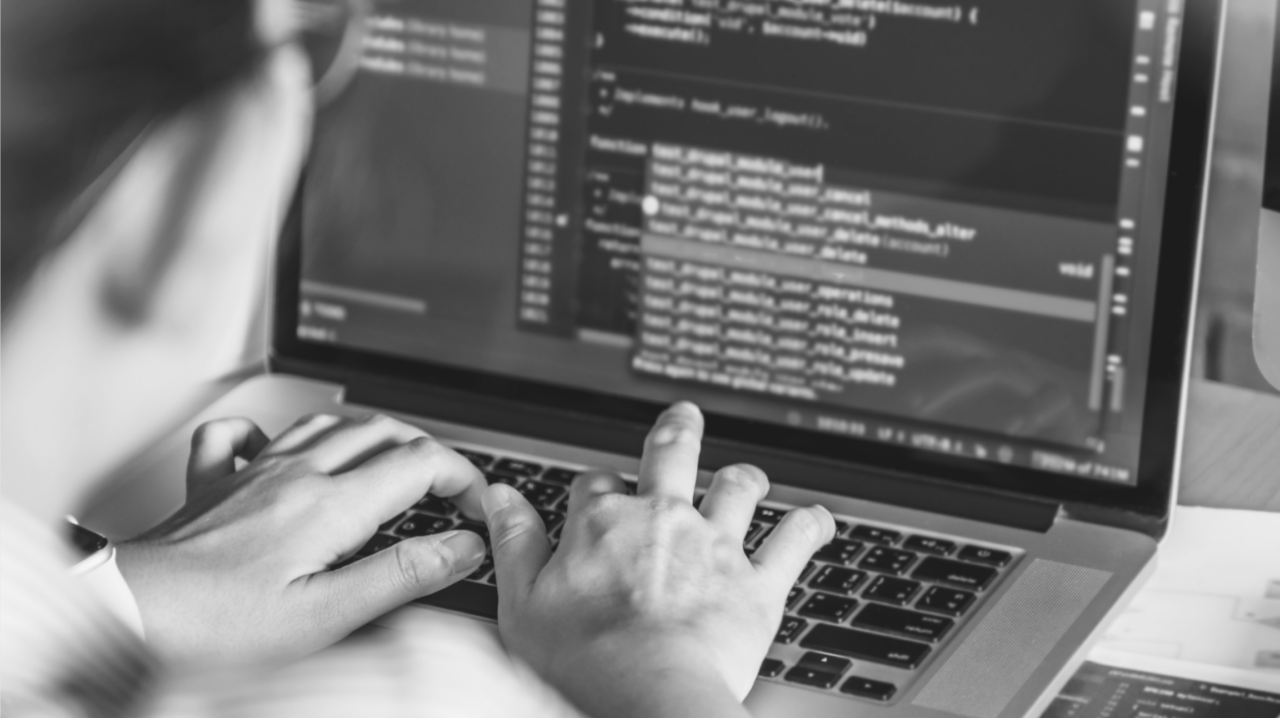
Enhancing AI Precision: Data Cleaning, Feature Engineering, and Labeling
Blog
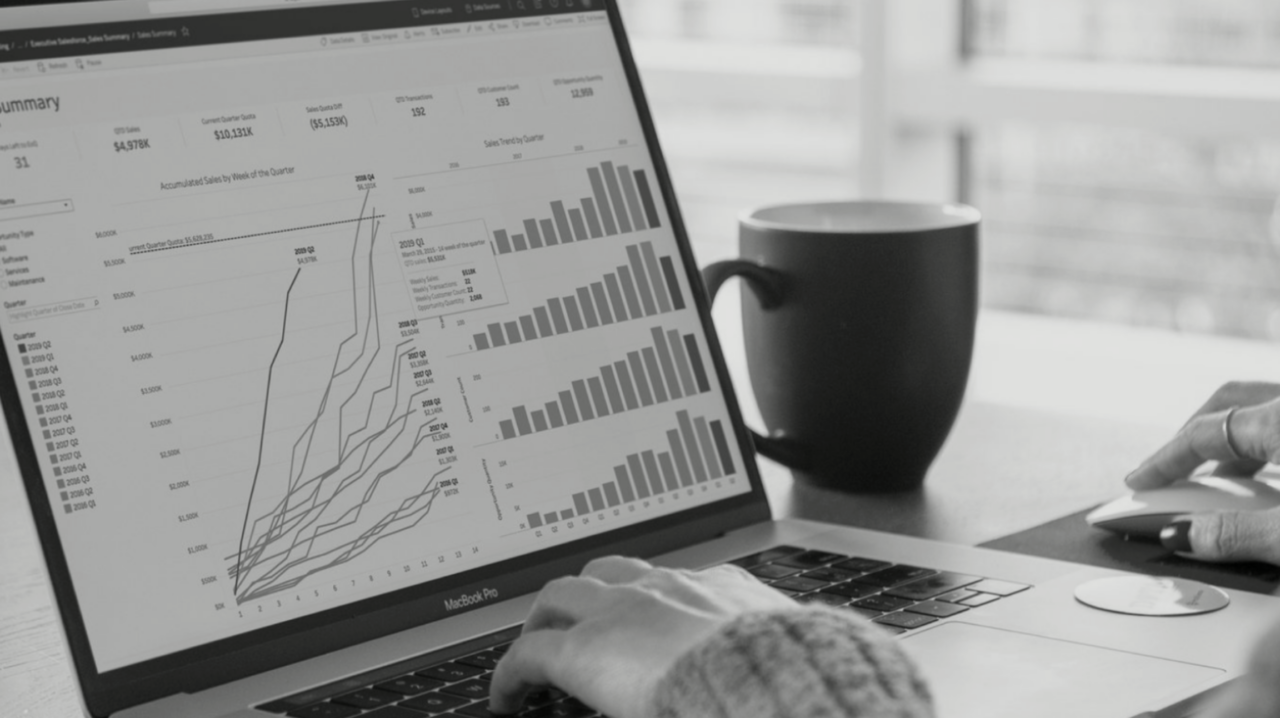
Unlocking the Power of AI: Why Data Readiness Matters
Blog
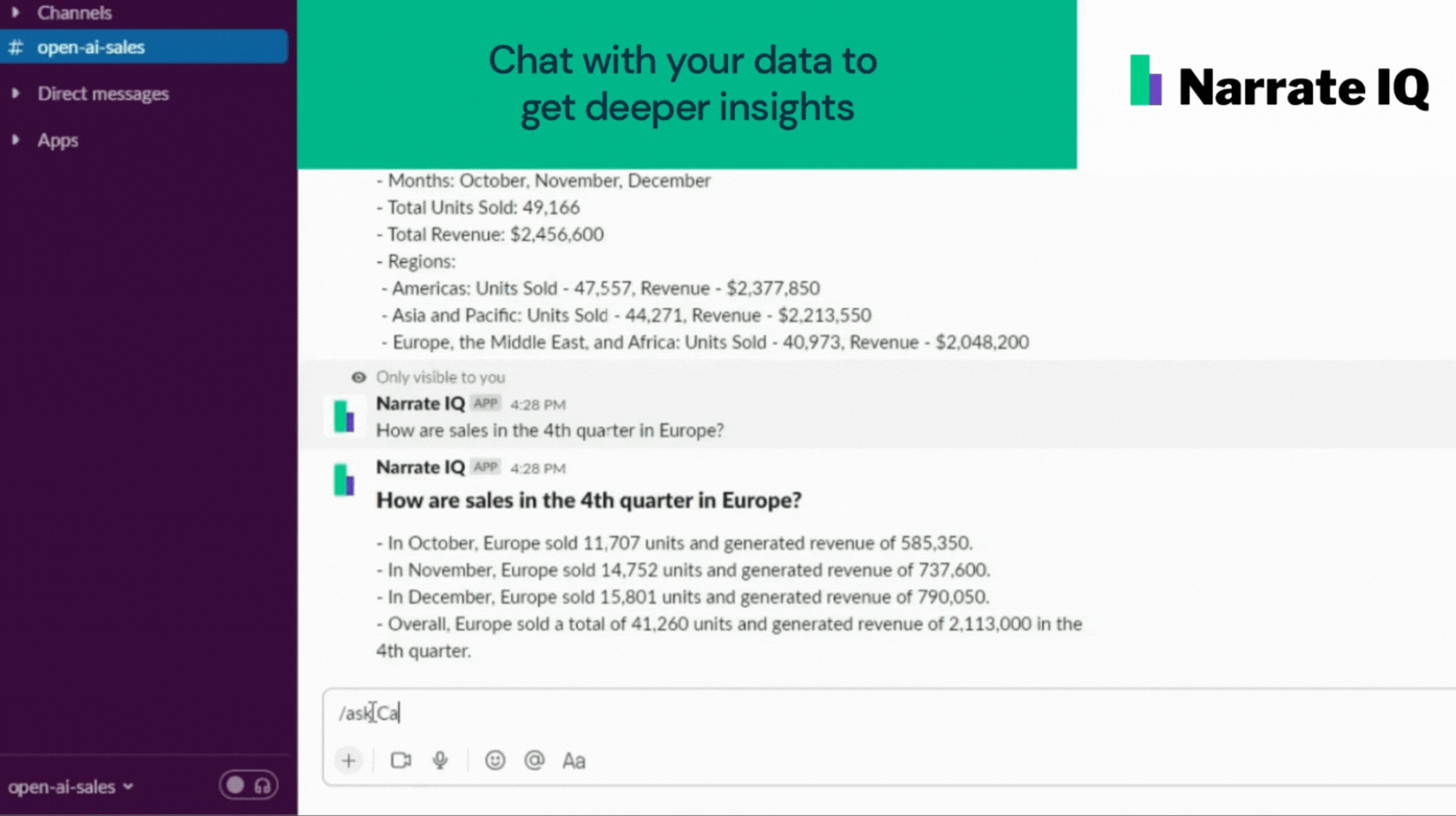
Narrate IQ: Delivering AI-Fueled Data Insights through Slack
Blog
Get Started
OneSix helps companies build the strategy, technology and teams they need to unlock the power of their data.